Ecosystem

Recently, the land surface changes have been accelerated due to forest destruction, urbanization and disasters, etc. A land-use and land-cover classification (LULC) map is required so that changing land surfaces time to time can be caught up on. EORC develops satellite high-order calibrated products required for the above, the reference database for classification and validation, and development of the classification algorithms makes great efforts to enhance the quality of these products through the partnership with universities and research organizations.
EORC has created comprehensive and high quality LULC map where JAXA's past, current and future satellite data items are effectively used and various sensors such as optical, microwave, active and passive, are combined.
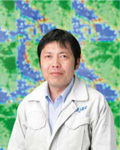
Takeo Tadono
Associate Senior Researcher
Earth Observation Research Center
Space Technology Directorate 1
Development of High Resolution Land Cover Map
EORC provides a 10m resolution land-use and land-cover map by using a high-order calibrated product mainly with the AVNIR-2 sensor aboard the Advanced Land Observing Satellite (ALOS) "DAICHI". This map is effectively used for the evaluation and assessment of agricultural land changes, etc.
Maintenance of Land Cover Classification Reference Database (Site-based dataset for Assessment of Changing Landcover by JAXA: SACLAJ)
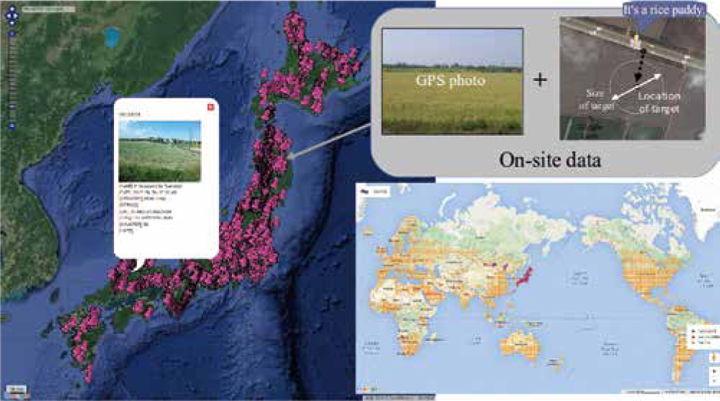
SACLAJ Data Base
EORC constructs a data base where on-ground information survey data (site photos, etc.) provided from web interfaces by researchers and students, and satellite images, aerial photographs and monitoring data are integrated to monitor long-term land surface changes.
Global 25m Resolution PALSAR-2/PALSAR Mosaic & Forest/Non-Forest(FNF) Map
This data set has been prepared with high-accuracy high-speed bulk processing analysis technology developed by EORC, applied to global data from the L-band Synthetic Aperture Radars (PALSAR and PALSAR-2) loaded on the Advanced Land Observing Satellites "DAICHI" (ALOS) and "DAICHI 2" (ALOS-2).
It has been confirmed that the classification accuracy of Forest/Non-Forest (FNF) maps is 84% or more of the reference data site photographs and high-resolution optic satellite images after comparison.